Hayes Process Macro - Model 7 - Moderated Mediation Analysis
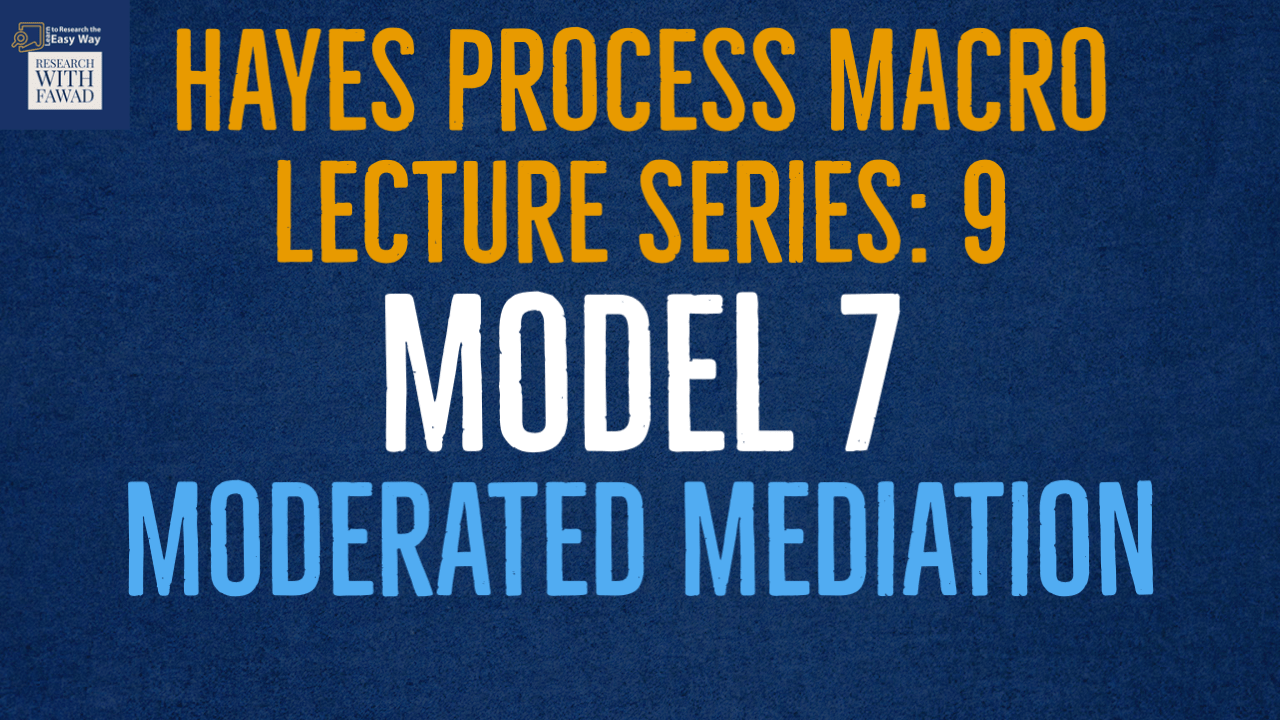
Hayes Process Macro - Lecture Series
The tutorial will guide on Model 7 of the Hayes Process Macro for Moderated Mediation.
Model 7
- Example Variables: 1 predictor X, 1 Mediators M, 1 Moderator W, and 1 Outcome Y
- The variables are continuous.
- Note: Model 7 allows up to 10 mediators operating in parallel.
Example
- In more complex models, you may have a mediation model that also has a moderator on one of the indirect paths.
- Not only are you trying to determine if mediation is present, but you are also trying to see if a moderator is changing the strength of the indirect effect.
- Let’s look at our moderation example, We are going to examine if the moderator is influencing the indirect effect. Our simple mediation test will be if Organizational Commitment leads to Organizational Performance through Organizational Learning.
- Our moderator will be the construct of Role Ambiguity, which negatively influences the relationship from Organizational Commitment to Organizational Performance. See the graphical representation that follows.
How to Run
- Step 1: Analyze -> Regression -> Process v4.0 by Andrew F. Hayes
- Step 2: Put in the Required Variables. In this case,
- Y Variable (Dependent Variable) is OP
- X Variable (Independent Variable) is OC
- Mediator is OL
- Moderator is RA
- Model Number is 7
Step 3: Select Option Button, and Choose the options as shown in the figure below and then Press Continue
Output Interpretation - Basic Summary
- Description of Model along with the different variables where Y is Dependent Variable, X is Independent Variable, M is the Mediating Variable, and W is the Moderator.
Output Interpretation - Model Summary
- Model Summary, provides summary of the model with R, R-Sq, F Statistics, and P value for the overall model.
- Model summary for the outcome variable (OL) is presented.
- OC has a significant impact on OL (b = 0.5015, t = 10.9201, p < 0.001).
- RA has a significant impact on OL (b = -0.0952, t = -2.2389, p = 0.0258).
- Int_1 (OC * RA) has a significant impact on OL (b = -.1370, t = -4.0265, p = 0.001).
Johnson-Neyman intervals and simple slopes analysis
- Johnson-Neyman interval tells you the range of values of the moderator in which the slope of the predictor is significant vs. nonsignificant at a specified alpha level
- In simple terms Johnson-Neyman identifies regions in the range of the moderator variable where the effect of the focal predictor on the outcome is statistically significant and not significant.
Visualizing Effects
- Next part of the Hayes Process Macro output is about Data Visualization.
- You will see the following code, to get the graph, copy the code by double clicking on the output.
- Next, Click File menu, New, and then Syntax.
- Paste the Data Visualization code that you copied. Now, Click the Run Selection button.
- You will have the graph with the main output, at the bottom of the Hayes Process Macro original output. (I have simply connected the dots, the next section presents the interpretation.
Interpretation of Graph
- The graph shows a steeper gradient for low and average role ambiguity. The impact of Commitment on Organizational Learning (OL) is much stronger at low and average role ambiguity. However, at higher role ambiguity, the line tends to straighten, this shows that at higher role ambiguity, the increase in OC does not lead to similar change in the OL.
- Next, following table presented the effects at different level of the moderator.
- In conclusion, higher role ambiguity weakens the impact of OC on OL.
Output Interpretation
- Model summary for the outcome variable (OP) is presented.
- OC has a significant impact on OP (b = 0.4040, t = 8.3680, p < 0.001).
- OL has a significant impact on OP (b = 0.4113, t = 8.4173, p < 0.001).
Direct and Indirect Effects
- This is good initial information, but we still do not know about the indirect effect or how the moderator influences the indirect effect. For that, We need to look into DIRECT AND INDIRECT EFFECTS OF X ON Y.
- First, this section shows the Direct effect of X on Y
- Next, it provides for Conditional indirect effects of X on Y.
- The conditional indirect effects shows that the indirect effect is high at low RA, reduced at average RA, and further reduced at Higher RA. However, the conditional indirect effects are significant at the three levels.
- The indirect effect in the presence of the moderator (At Mean Level) is .206, and per the bootstrap, that is within the confidence interval at a p < .05.
- You will also see the indirect effects when we probed the interaction at one standard deviation below and above the mean. The results show than when the moderator is low, the indirect effect is .283, which is significant. Conversely, when the moderator is high, the indirect effect is .129, which is still significant as well. But the effect is reduced.
Index of Moderated Mediation
- The last test we need to assess is if the construct of Role Ambiguity is significantly moderating the indirect effect.
- We need to assess if the indirect effect is being moderated. This is assessed by the index of moderated mediation value.
- This analysis examines if the slope is significantly different than zero, which indicates that moderated mediation is taking place.
- We can also see that the index of moderated mediation is significant. Thus, we can conclude that the indirect effect is moderated by Role Ambiguity.
Reporting Moderated Mediation Analysis
Following table can be utilized to presented moderated mediation analysis results. The scholar(s) may develop different hypotheses based on the moderated mediation model including direct and indirect hypotheses.
For example, i am presenting a sample write-up for moderated mediation hypothesis.
- Hypothesis H1 suggested that the indirect effect of Organizational Commitment on Organizational Performance through Organizational Learning will be moderated by Role Ambiguity. H1 was supported as the index of moderated mediation (index = -0.056, 95% CI = [-0.094/-0.019]) is significant since the 95% CI does not include zero.
Video Tutorial
Additional Hayes Process Macro Tutorials
- Hayes Process Macro – Model 1 – Moderation with Continuous Moderator, IV and DV
- Hayes Process Macro – Model 1b – Moderation with Categorical Moderator and Continuous IV and DV
- Hayes Process Macro – Model 2 – Moderation with Two Continuous Moderators
- Hayes Process Macro – Model 4 – Mediation Analysis
- Hayes Process Macro – Model 4 – Mediation Analysis with Multiple Mediators
- Hayes Process Macro – Model 5 – Multiple Mediators and 1 Moderator
- Hayes Process Macro – Model 6 – Serial Mediation
- Hayes Process Macro – What is Hayes Process Macro and How to Install it