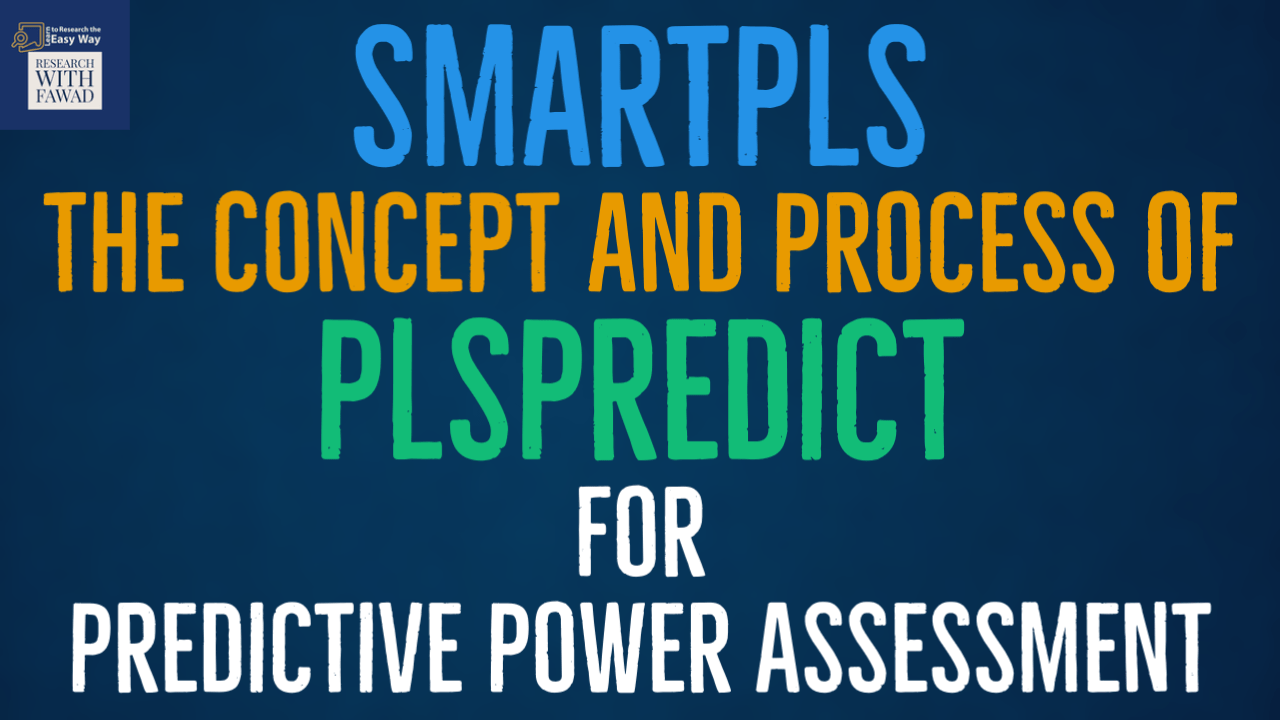
Learn to assess Predictive Power in PLS-SEM
The Concept and Process of Predictive Power Assessment using PLSPredict in SmartPLS3
The Concept and Process of Predictive Power Assessment using PLSPredict in SmartPLS3
If all indicators in the PLS-SEM analysis have lower RMSE (or MAE) value compared to the naïve LM benchmark, the model has high predictive power.
If the majority (or the same number) of indicators in the PLS-SEM analysis yields smaller prediction errors compared to the LM, this indicates a medium predictive power.
If a minority of the dependent construct’s indicators produce lower PLS-SEM prediction errors compared to the naïve LM benchmark, this indicates the model has low predictive power.
If the PLS-SEM analysis (compared to the LM) yields lower prediction errors in terms of the RMSE (or the MAE) for none of the indicators, this indicates the model lacks predictive power.
If all indicators in the PLS-SEM analysis have lower RMSE (or MAE) value compared to the naïve LM benchmark, the model has high predictive power.
If the majority (or the same number) of indicators in the PLS-SEM analysis yields smaller prediction errors compared to the LM, this indicates a medium predictive power.
If a minority of the dependent construct’s indicators produce lower PLS-SEM prediction errors compared to the naïve LM benchmark, this indicates the model has low predictive power.
If the PLS-SEM analysis (compared to the LM) yields lower prediction errors in terms of the RMSE (or the MAE) for none of the indicators, this indicates the model lacks predictive power.