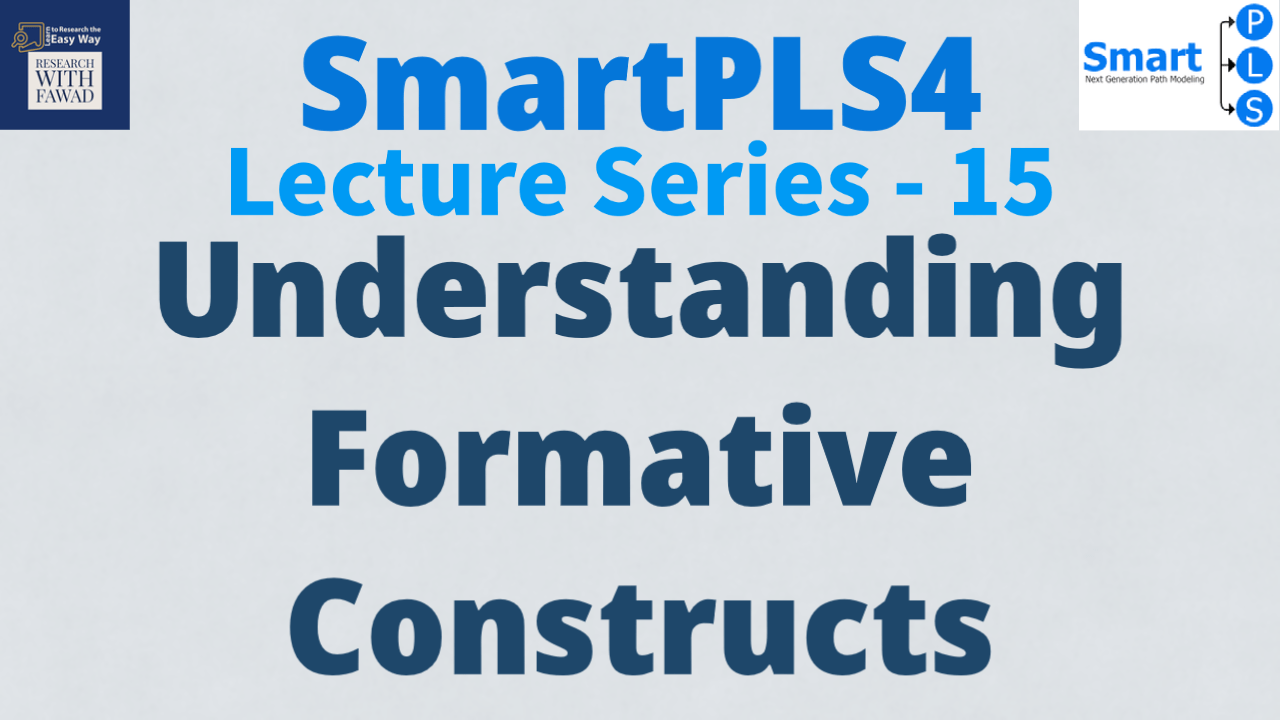
Understanding Concept of Formative Construct in SmartPLS4?
For Complete Step by Step SmartPLS4 Tutorial Playlist, Click Here
For Complete Step by Step SmartPLS4 Tutorial Playlist, Click Here
PLS-SEM is the preferred approach when formatively specified constructs are included in the PLS path model. In this session, I discuss the key steps for evaluating formative measurement models (See Fig). Relevant criteria include the assessment of (1) convergent validity, (2) indicator collinearity, and (3) statistical significance and relevance of the indicator weights.
In the following, key criteria and their thresholds are presented to validate a formative construct.
In formative measurement model evaluation, convergent validity refers to the degree to which the formatively specified construct correlates with an alternative reflectively measured variable(s) of the same concept. Originally proposed by Chin (1998), the procedure is referred to as redundancy analysis. To execute this procedure for determining convergent validity, researchers must plan ahead in the research design stage by including an alternative measure of the formatively measured construct in their questionnaire. Cheah, Sarstedt, Ringle, Ramayah, and Ting (2018) show that a global single item, which captures the essence of the construct under consideration, is generally sufficient as an alternative measure. Hair et al. (2022) suggest the correlation of the formatively measured construct with the reflectively measured item(s) should be 0.708 or higher, which implies that the construct explains (more than) 50% of the alternative measure’s variance.